World-wide experiments have been conducted to understand the distinct relationships among various genes. However, it remains a challenge to identify the genomic causes and effects directly from the data, especially within a network. It’s the classic chicken and egg question: Which comes first, the chicken or the egg? In other words, how do you know which genes regulate which other genes?
Correlation between the expression of two genes is symmetrical. Therefore, scientists cannot infer which of the two genes is the regulator and which is the target. Similar levels of correlation can arise from different causal mechanisms. For example, between two genes with correlated expression levels, it is plausible that one gene regulates the other gene; it is also plausible that they do not regulate each other directly, but are regulated by a common genetic variant.
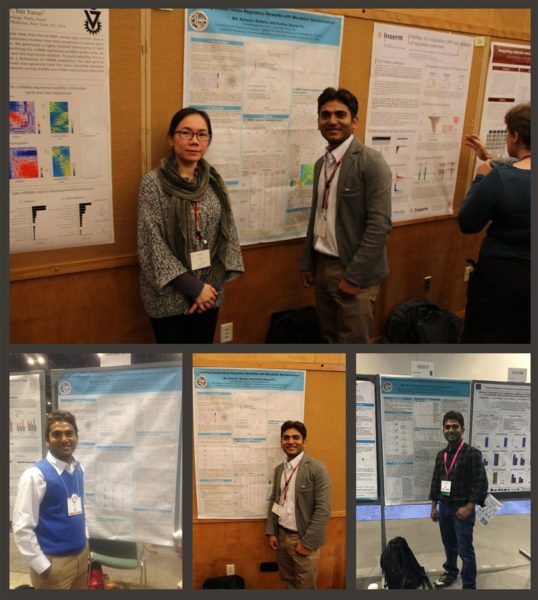
Audrey Fu, Assistant Professor in the Department of Statistical Science, and Postdoctoral Researcher Md. Bahadur Badsha, recently published a paper introducing a novel machine learning algorithm. “Our new method, namely the MRPC algorithm, can tease apart which correlation may suggest causality and which correlation is just indirect association through many other genes,” said Fu.
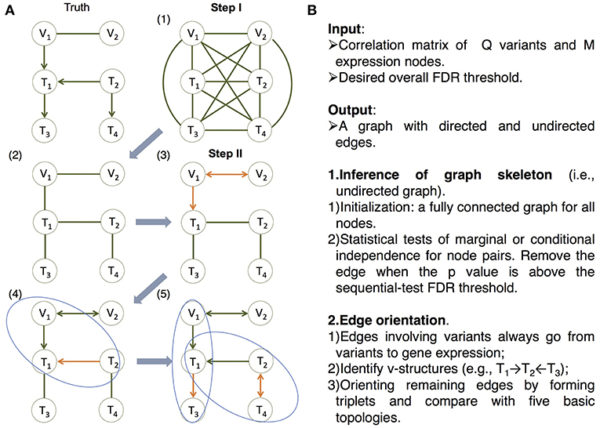