The CMCI Reproducibility in Sciences working group, or SciRep for short, has been meeting since the fall of 2015. Today, their most recent publication, “Scientific discovery in a model-centric framework: Reproducibility, innovation, and epistemic diversity,” was published in PLOS ONE. Congratulations!
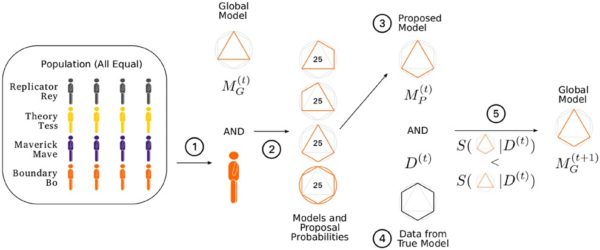
The American Council on Science and Health also picked up the story with their article, “Reconsidering The ‘Replication Crisis’ In Science.”
The following article was written by Leigh Cooper, U of I Science and Content Writer.
U of I Study Finds Scientific Reproducibility Does Not Equate to Scientific Truth
MOSCOW, Idaho — May 15, 2019 — Reproducible scientific results are not always true and true scientific results are not always reproducible, according to a mathematical model produced by University of Idaho researchers. Their study, which simulates the search for that scientific truth, was published today, May 15, in the journal PLOS ONE.
Independent confirmation of scientific results — known as reproducibility — lends credibility to a researcher’s conclusion. But researchers have found the results of many well-known science experiments cannot be reproduced, an issue referred to as a “replication crisis.”
“Over the last decade, people have focused on trying to find remedies for the ‘replication crisis,’” said Berna Devezer, lead author of the study and U of I associate professor of marketing in the College of Business and Economics . “But proposals for remedies are being accepted and implemented too fast without solid justifications to support them. We need a better theoretical understanding of how science operates before we can provide reliable remedies for the right problems. Our model is a framework for studying science.”
Devezer and her colleagues investigated the relationship between reproducibility and the discovery of scientific truths by building a mathematical model that represents a scientific community working toward finding a scientific truth. In each simulation, the scientists are asked to identify the shape of a specific polygon.
The modeled scientific community included multiple scientist types, each with a different research strategy, such as performing highly innovative experiments or simple replication experiments. Devezer and her colleagues studied whether factors like the makeup of the community, the complexity of the polygon and the rate of reproducibility influenced how fast the community settled on the true polygon shape as the scientific consensus and the persistence of the true polygon shape as the scientific consensus.
Within the model, the rate of reproducibility did not always correlate with the probability of identifying the truth, how fast the community identified the truth and whether the community stuck with the truth once they identified it. These findings indicate reproducible results are not synonymous with finding the truth, Devezer said.
Compared to other research strategies, highly innovative research tactics resulted in a quicker discovery of the truth. According to the study, a diversity of research strategies protected against ineffective research approaches and optimized desirable aspects of the scientific process.
Variables including the makeup of the community and complexity of the true polygon influenced the speed scientists discovered the truth and persistence of that truth, suggesting the validity of scientific results should not be automatically blamed on questionable research practices or problematic incentives, Devezer said. Both have been pointed to as drivers of the “replication crisis.”
“We found that, within the model, some research strategies that lead to reproducible results could actually slow down the scientific process, meaning reproducibility may not always be the best — or at least the only — indicator of good science,” said Erkan Buzbas , U of I assistant professor in the College of Science , Department of Statistical Science and a co-author on the paper. “Insisting on reproducibility as the only criterion might have undesirable consequences for scientific progress.”